As Artificial Intelligence (AI) continues to advance, it brings both immense potential and complex challenges to the field of cybersecurity. Let’s delve into what you need to know about this intersection:
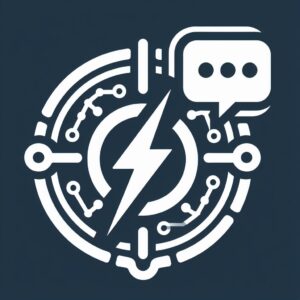
1. The Transformative Power of AI:
- AI has permeated virtually every industry, revolutionizing processes and driving innovation.
- Tools like ChatGPT and Alphabet’s Bard showcase AI’s generative capabilities, enabling creativity, improved customer service, and better decision-making.
2. The Dual Nature of AI in Cybersecurity:
- Threats: As AI evolves, so do cyber threats. Skilled cybercriminals can manipulate AI tools to their advantage.
- Skills Gap: The world faces a shortage of 3.4 million cybersecurity professionals, making it challenging to defend against AI-driven attacks.
- Responsibility: AI must be harnessed responsibly and securely to avoid unintended consequences.
3. Sophisticated Attacks:
- Spear-Phishing: Imagine a cybercriminal using AI to craft highly personalized spear-phishing messages. These messages blend seamlessly with an organization’s internal communication style, deceiving even vigilant employees.
- Deepfakes: AI-generated deepfake voices can impersonate high-ranking executives, potentially leading to unauthorized fund transfers.
4. Data Tampering:
- Attackers can use AI to manipulate system data, creating enticing yet entirely fabricated stock portfolios.
- Traditional security systems often struggle against such sophisticated attacks.
5. AI as a Solution:
- Real-Time Monitoring: AI algorithms analyze massive data to detect patterns indicative of cyber threats. It scans networks for weaknesses and prevents common attacks.
- Automated Incident Response: AI streamlines threat hunting and improves cybersecurity by analyzing large datasets.
- Behavioral Analysis: AI monitors and analyzes behavior patterns, enhancing threat detection.
6. The Future Landscape:
- AI extends our ability to shield ourselves from online threats.
- By leveraging AI, we can proactively defend against cyber attacks, automate responses, and stay ahead of evolving threats.
What are some AI-driven cybersecurity tools?
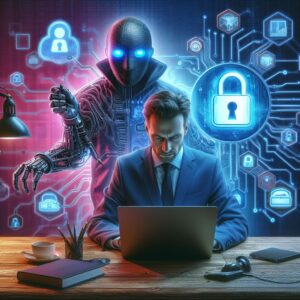
Certainly! Here are some AI-driven cybersecurity tools that play a crucial role in safeguarding digital environments:
- Endpoint Protection with AI:
- Behavioral Analysis: These tools monitor endpoints (devices like computers, servers, and mobile devices) for unusual behavior. AI algorithms detect patterns associated with malware or unauthorized access.
- Predictive Analysis: By analyzing historical data, these tools predict potential threats and take preventive measures.
- Network Intrusion Detection Systems (NIDS):
- AI-enhanced NIDS: These systems use machine learning to identify abnormal network traffic. They learn from patterns and anomalies, raising alerts when suspicious activity occurs.
- Deep Packet Inspection: AI-powered NIDS analyze packet-level data to detect threats, such as zero-day vulnerabilities or advanced persistent threats.
- User and Entity Behavior Analytics (UEBA):
- User Profiling: UEBA tools build profiles of users and entities (such as applications and devices). They detect deviations from normal behavior, flagging potential insider threats.
- Anomaly Detection: UEBA leverages AI to identify unusual activities, such as excessive data access or unauthorized logins.
- Security Information and Event Management (SIEM):
- Log Analysis: SIEM tools collect and analyze logs from various sources (firewalls, servers, etc.). AI helps correlate events, detect patterns, and prioritize incidents.
- Threat Intelligence Integration: AI-enhanced SIEM integrates threat intelligence feeds, enhancing threat detection accuracy.
- Machine Learning-based Antivirus:
- Dynamic Models: Traditional signature-based antivirus struggles with new threats. ML-based antivirus adapts by learning from evolving attack patterns.
- Zero-Day Detection: ML algorithms identify zero-day vulnerabilities by analyzing file behavior.
- Automated Threat Hunting:
- AI-driven Threat Hunting: These tools proactively search for threats within an organization’s network. They analyze data, identify anomalies, and uncover hidden threats.
- Security Orchestration, Automation, and Response (SOAR):
- Workflow Automation: SOAR platforms automate incident response workflows. AI assists in decision-making, prioritizing incidents, and executing predefined actions.
- Playbook Execution: SOAR executes predefined playbooks based on AI analysis, reducing response time.
- Adaptive Authentication:
- Risk-based Authentication: AI assesses user behavior, device information, and context to determine risk levels. It prompts additional authentication steps when needed.
- Cloud Security with AI:
- Anomaly Detection in Cloud Traffic: AI monitors cloud traffic for unusual patterns, ensuring data security.
- Configuration Monitoring: AI scans cloud configurations for misconfigurations that could lead to vulnerabilities.
- Threat Intelligence Platforms (TIP):
- Automated Threat Feeds: TIPs aggregate threat intelligence from various sources. AI processes this data, providing actionable insights.
- Threat Scoring: AI assigns risk scores to threats, aiding prioritization.
Remember that while AI enhances cybersecurity, it’s essential to combine it with human expertise. Cybersecurity professionals play a critical role in configuring, monitoring, and fine-tuning these tools to stay ahead of evolving threats.
What are the limitations of AI-driven cybersecurity?
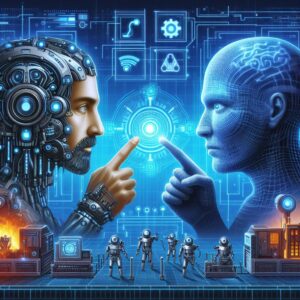
Certainly! AI-driven cybersecurity has made significant strides, but it also faces several limitations. Let’s explore them:
1. False Positives:
- AI algorithms are not infallible. They occasionally flag benign activities as potentially malicious, leading to false positives.
- These false alarms can consume valuable time and resources for security teams.
2. Data Privacy Concerns:
- AI processes vast amounts of data. If not adequately secured, there’s a risk of data privacy violations.
- Safeguarding sensitive information is crucial to prevent unintended leaks.
3. Ambiguity Handling:
- Current AI tools struggle with situations where there is no definitive answer.
- Unlike human brains, AI lacks the ability to navigate ambiguity effectively¹[3].
4. Limited Knowledge:
- AI systems heavily rely on their trained data sets. They may be impotent when faced with novel or intricate threats beyond their recognized realm²[5].
5. Dependency on Data:
- AI engines, whether for good or malicious purposes, are constrained by the data they ingest.
- Lack of diverse and comprehensive training data can limit their effectiveness.
6. Adversarial Attacks:
- Sophisticated adversaries can exploit AI vulnerabilities. They may manipulate models or introduce adversarial inputs.
- AI defenses need to be robust against such attacks.
7. Human Expertise Still Essential:
- While AI augments cybersecurity, human expertise remains critical.
- Security professionals interpret context, adapt strategies, and make judgment calls that AI alone cannot replicate.
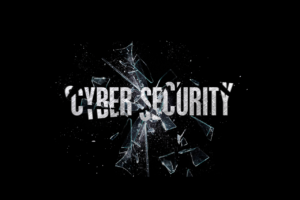
Source: Conversation with Bing, 4/28/2024